If there is one area where artificial intelligence (AI) is making an impact in radiology, it is in breast radiology, particularly in screening. Due to capacity shortages, AI has already become part of regular screening programs in several countries, partially taking over the role of one of the two readers. What are the latest developments in AI for breast radiology and what are companies currently working on?
The players
Since the advent of deep learning around 2015, AI companies in radiology have emerged rapidly, including in breast radiology. Screening programs with a high volume of imaging are making breast radiology an attractive area for developers, entrepreneurs and investors all over the world. Currently, there are fifteen companies with over twenty AI products available on the European market, all CE-marked and clinically approved for their intended use.
AI solutions for detecting breast cancer on mammograms are now familiar to most radiologists. Companies are emerging from all over the world, as shown in Figure 1. While the number of AI companies is still growing in many medical disciplines, the situation in radiology is different. In fact, the number might even be decreasing as companies withdraw from the market or merge. For example, the recent news of the acquisition of Kheiron Medical Technologies by DeepHealth and earlier this year, Korean company Lunit acquired New Zealand-based Volpara. Despite this, the range of products remains broad and offers a wide selection.
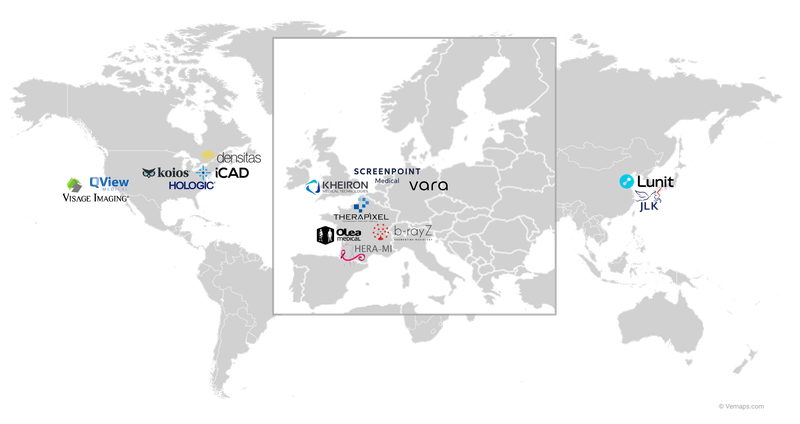
Figure 1: Worldmap with the head offices of the vendors that have breast radiology AI solutions. [Volpara was no longer included separately because of the acquisition.]
AI offerings
Figure 2 shows an overview of which companies provide AI applications in breast radiology. Most products assist in detecting abnormalities in mammograms and digital tomosynthesis, although the methods vary, ranging from annotations to risk scores, trained on the radiologist's BI-RADS or pathology data, image enhancement, and triage. Additionally, there are a few applications for MRI and ultrasound.
AI can assist not only radiologists but also technicians and quality assurance organizations with positioning techniques. Efforts are underway, but such applications are challenging. The software needs to recognize various structures (e.g., pectoralis muscle, nipple, breast-abdomen junction), and there’s a level of subjectivity in determining when the acquisition is “good enough.” Both factors make creating a suitable AI product challenging. AI for positioning is often a secondary development focus for companies, while AI for cancer detection is a priority. This also seems to be the case for the implementation of AI applications in breast radiology.
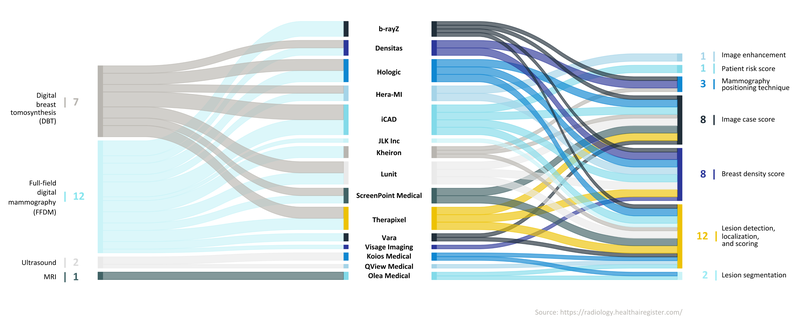
Figure 2: Companies offering CE-certified AI products for breast radiology. On the left, the modalities for which the companies provide a solution are shown. On the right, the functionalities they offer are listed. Some functionalities are combined into a single product offering. [This figure was created by Marta Pinto.]
Screening in practice
It was hard to miss last summer: the MASAI study results spread from The Lancet to newspapers like The Washington Post. It was the first randomized clinical trial on the impact of AI in breast cancer screening. Initial results from this Swedish study showed that, thanks to AI, cancer was detected in 6 out of every 1,000 women instead of 5, with a nearly identical referral rate and 40% less reading time. More recent results presented at the EUSOBI annual meeting and European Congress of Radiology (ECR) confirmed these positive impacts. Many are eagerly awaiting data on AI’s impact on interval cancers.
Sweden isn’t the only country taking AI in screening seriously; Denmark already uses it in the Copenhagen region’s regular program, with two of its four other regions preparing for implementation. Australia and Iceland have also selected its providers and are moving towards implementation. Prospective study results are also emerging from England, Scotland, Germany, Spain, and Norway.
Your “own” model
As AI continues to become more widely adopted, the technology continues to evolve as well. An interesting advancement is the first continuous learning product. Swiss company b-rayZ offers each healthcare organization its own model, where authorized users can provide feedback on AI’s findings, allowing the “personal” model to learn in the background from these data points. They deviate from convention by training the algorithm on radiologists’ assessments rather than pathology data, a bold choice in cancer detection, yet possibly interesting when applied to positioning techniques. Given subjectivity and varying criteria, a personalized model for a healthcare organization or country could be beneficial. One thing is certain: this is an innovative approach, unlike anything yet seen in radiological AI applications.
What’s next?
Currently, most AI products analyze images from a single screening timepoint, lacking access to prior images that radiologists often rely on for comprehensive assessment. This is expected to change soon. Several providers have announced plans to incorporate prior images into assessments. Expectations are high: the accuracy of these products should improve, resulting in fewer false positives and better detection.
Additionally, risk-based screening is gaining attention. Currently, only the American company iCAD offers a CE-marked product that provides a risk score indicating the likelihood of a screening participant developing breast cancer within a certain number of years. This product factors in not only imaging data but also breast density and age. It is likely that they will not be the only ones for long, as recent studies suggest that other companies are quietly working on similar developments.
Conclusion
The future of AI in breast radiology looks promising. Although the market for providers appears to have stabilized, competition remains fierce, and providers are not idle. After years of development and retrospective studies, we now see the first real-world results coming in. While implementation approaches may vary across healthcare organizations and countries, the trend is clear: AI will play an integral role in improving breast cancer diagnostics and screening efficiency. It is now up to hospitals, healthcare systems, and policymakers to responsibly and effectively integrate this technology to maximize benefits for patients and screening participants. The question is no longer if, but how and when AI will be fully integrated into the routine practice of breast radiology.
Author: Kicky van Leeuwen, founder of Health AI Register and managing partner of Romion Health, also serving as project leader for AI in breast cancer screening at the Dutch National Institute for Public Health and the Environment (RIVM). A variation of this article named 'Hoe AI de mammaradiologie verandert' was previously published in Dutch in the Memorad.