Navigating the landscape of AI tools in neuroradiology can feel like a daunting task. With numerous vendors offering a variety of products, each with its own unique functionalities and applications, it is easy to get lost in the chaos. While resources like our Health AI Register provides some guidance, we want to take things a step further.
In this blog, we are diving deep into a specific and impactful condition: Intracranial Hemorrhage (ICH). Recent studies suggest that AI tools have the potential to significantly enhance both the quality and efficiency of ICH care. With this in mind, we have set out to create a comprehensive overview of the currently available commercial AI products designed for managing intracranial hemorrhages, along with their available evidence.
Join us as we explore how AI is having an impact on the field of ICH management, offering insights into the latest advancements and helping you make informed decisions in this rapidly evolving area.
Here is what we did
We started by compiling a comprehensive list of all the available commercial AI products designed to aid in ICH management by using the Health AI Register and its various filters.
This resulted in the following AI tools:
- Annalise Enterprise CTB – Annalise.AI
- BrainScan CT – BrainScan Inc.
- CINA-ICH – Avicenna.AI
- DeepCT – Deep01 Limited
- Deepstroke – Methinks
- Intracranial Hemorrhage (briefcase) – Aidoc Medical
- JBS-04K – JLK Inc.
- qER – Qure.AI
- Rapid ICH – RapidAI
- StrokeViewer – NicoLab
- Viz ICH – Viz.AI
Most of the products are designed to streamline the process by focusing on prioritization and triage. When new CT images are acquired and orchestrated to the PACS system, these tools automatically analyse them, sorting through the, sometimes massive, volume of images that come in. The AI algorithms detect ICH and send alerts to radiologists so they know exactly which images need their immediate attention. These functionalities have the potential to be game-changers for diagnostic accuracy and efficiency, but to what extent is this potential actually being realized?
To answer this question, we turned to the latest peer-reviewed literature that were linked to the specific products. You can find all the used studies listed on the product-pages of the Health AI Register (linked above) at the bottom of each page.
This is what we found
For this blog post, we reviewed 19 articles, with most of them evaluating the stand-alone diagnostic performance of these products, and the results were impressive. Sensitivity ranged from 87.2% (Seyam et al., 2022) to 97.84% (Sreekrishnan et al., 2023), while specificity varied between 84.4% (Eldaya et al., 2022) and 99.52% (Chien et al., 2022; Sreekrishnan et al., 2023). For those products that handle segmentation quantification, two studies focused on volumetric analysis reported an intraclass correlation coefficient (ICC) of 0.96 (Hillal et al., 2023) and a correlation coefficient of 0.983 (Heit et al., 2021).
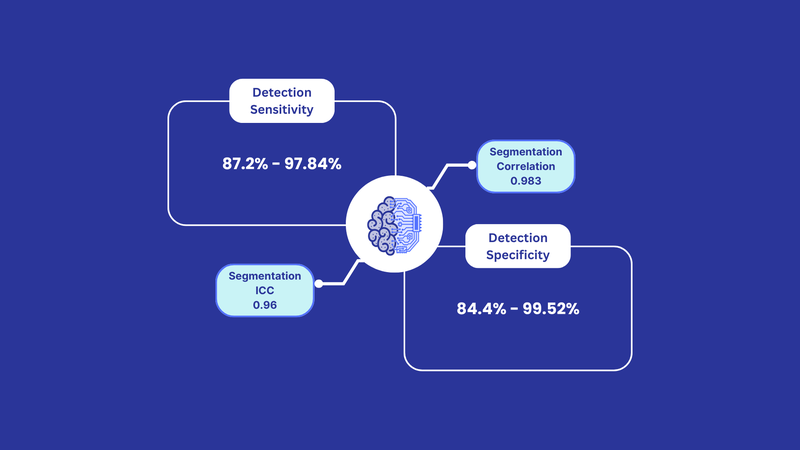
Reported performance of commercially available Al solutions for ICH detection and segmentation.
However, what we really want to know is the impact on clinical practice, and here, the results are less straightforward. While there is a noticeable improvement in diagnostic performance when radiologists use AI tools to aid in their decision (Buchlak et al., 2024; Chekmeyan et al., 2023; Eldaya et al., 2022), the efficiency gains are more ambiguous. Comparisons between ICH and non-ICH cases (Ginat, 2021; O’Neill et al., 2021; Seyam et al., 2022), as well as pre-AI versus post-AI scenarios (O’Neill et al., 2021; Seyam et al., 2022), show mixed results. On a brighter note, one supposed benefit is the reduction in patient length of stay (Chien et al., 2022; Petry et al., 2022), which stands out as a particularly promising outcome for now. For easier reference, we have summarized these findings in the table below.
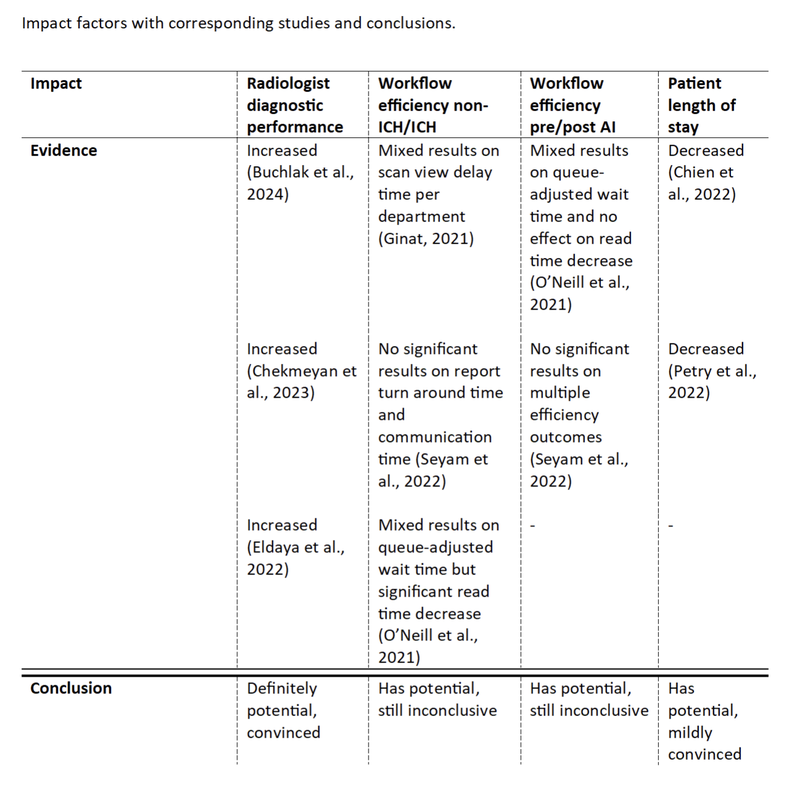
Other interesting insights
When we examined the articles more closely, we discovered some intriguing insights. The distribution of articles, efficacy levels, and geographical representation revealed several patterns and biases.
The first interesting aspect we observed was a significant skew in the distribution of products the articles were about. Out of nineteen articles, one particular AI product dominated the scene, claiming eleven of them. In contrast, other products were scarcely represented, with some appearing in just one article or none at all. This disparity raises an eyebrow, especially given the vendors' frequent assertions that their products are backed by scientific literature.
We also looked at what type of evidence the paper is bringing to the table, described by the level of efficacy. This highlighted the focus on diagnostic accuracy (level 2) which includes stand-alone performance studies.
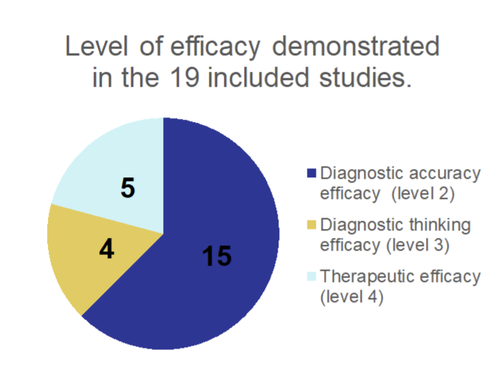
The emphasis on diagnostic accuracy is understandable, as these foundational studies are essential before higher-level efficacy research can proceed. However, the scarcity of higher-level studies suggests a gap that needs to be addressed for a more comprehensive understanding of the real world impact these AI products have.
Another intriguing find was the geographical bias in the data sources. Despite all the AI products being CE marked, only two out of the nineteen articles explicitly mentioned they drew on data from European countries.
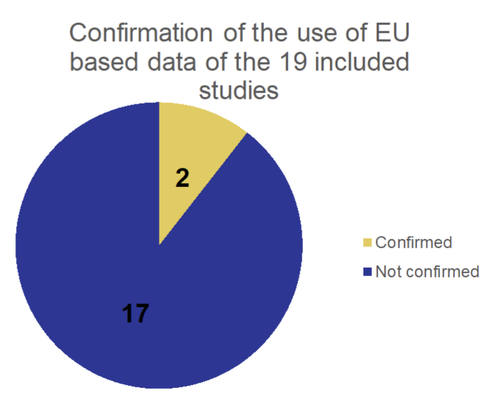
This underrepresentation of European sources poses challenges in reaching a global consensus on the effectiveness and the generalizability of these products, which are crucial for their widespread implementation in clinical practice.
Concluding
It's clear that there's more beneath the surface when examining these publications, revealing both biases and areas for further investigation.
As we wrap up our exploration into AI products aiding in ICH management, a few crucial points stand out:
- The potential for AI products to revolutionize clinical practice in ICH management is promising but not yet conclusive on all the clinical impact factors.
- Issues with proving higher-level efficacy and the lack of comprehensive EU data remain significant hurdles.
- Many products lack the robust, independent research validation that their vendors often claim.
As we continue to advance in the field of AI in healthcare, it is essential to approach these products with a critical eye, ensuring that they are not only innovative but meet their promise when adopted in clinical practice. Only then can we fully harness their potential to make our healthcare system futureproof.
Curious to find out which AI solutions fits your clinical setting? Contact us here.
References
Buchlak (2024), Chekmeya (2023), Chien (2022), Eldaya (2022), Ginat (2021), Heit (2021), Hillal (2023), O’Neill (2021), Petry (2022), Seyam (2022), Sreekrishnan (2023).